Update on WP4 activities
Fraunhofer ISC in Würzburg hosted a joint workshop together with the IT University of Copenhagen (ITU) and the University of Tartu (UTARTU). During the visit, a diverse programme enabled guests to experience the existing robot-based automation platform first-hand. The progresses and achievement of specific goals were addressed in detail in an interactive and fruitful discussions. Subsystems of the plant were successfully tested, ITU team adapted their analysis algorithms, carried out extensive data collection and worked out efficient cleaning cycles for the in-house liquid/liquid extraction device managing a smooth integration of the API for the dosing equipment, which was a significant milestone. In addition, both acquired and self-built devices were successfully put into operation. UTARTU team members were extremely happy to actually sit at the devices and machines for which he has been programming the back-end for so long!
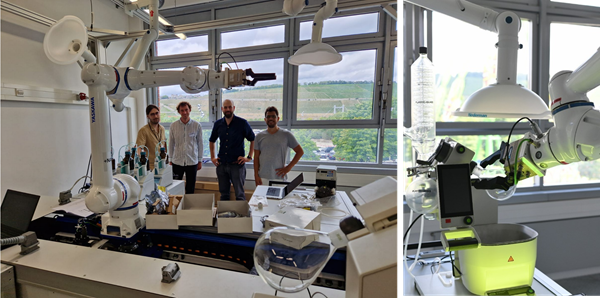
The careful preparation beforehand promoted a seamless effort integration. An unforgettable moment was the last cooperation day, when all colleagues worked dedicatedly on the plant!
Top downloaded paper: BIG-MAP Data Management Plan
The manuscript reporting the BIG-MAP Data Management Plan is a top downloaded article in Battery&Supercaps. This stresses how crucial research data management is and how BIG-MAP is pioneering a FAIR data infrastructure.
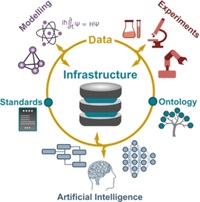
- New paper: Uncertainty-aware and explainable machine learning for early prediction of battery degradation trajectory (April 2023)
- Our autoregressive neural network predicts cell aging to accelerate the discovery of improved battery chemistries, while providing reliable uncertainty estimates and requiring minimal initial cycles. The model has a low Root Mean Square Error of only 106 cycles, and our explainability analysis suggests alignment with prior chemical insights.
- Full Description: Maximizing the lifespan of batteries is crucial in their design and development. However, accurately assessing a battery's lifespan can be time-consuming due to lengthy cycling experiments. By utilizing our autoregressive neural network to predict cell aging early on, we can shorten the duration of each experiment and expedite the discovery of improved battery chemistries.
Our deep learning model provides reliable uncertainty estimates, allowing for early experiment termination based on uncertainty, and requires only a minimal number of initial cycles to predict the battery's degradation trajectory. The model has an RMSE of only 106 cycles for the projected lifespan. Furthermore, our explainability analysis suggests that the model aligns with prior chemical insights, despite not being trained for this purpose.
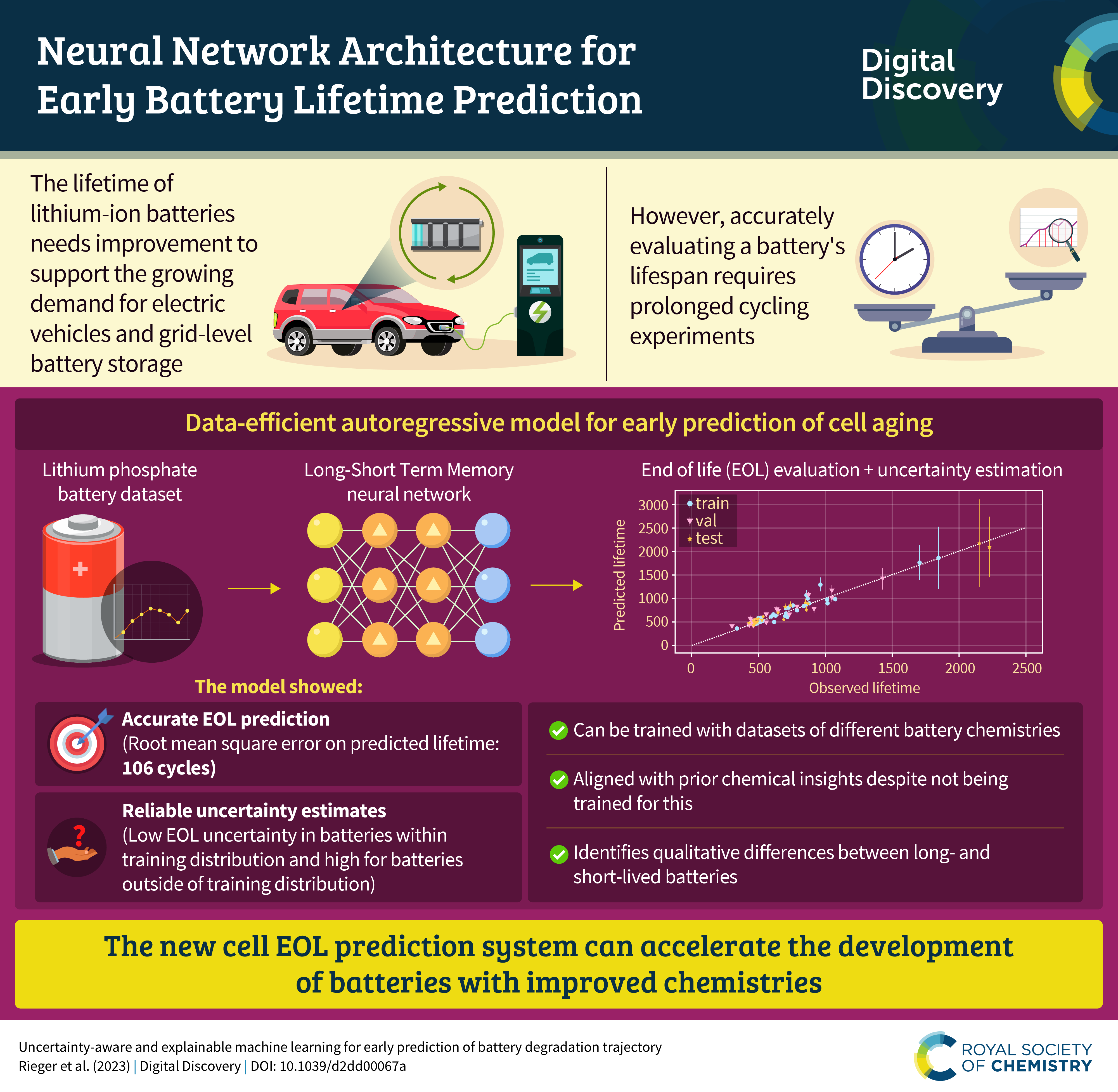
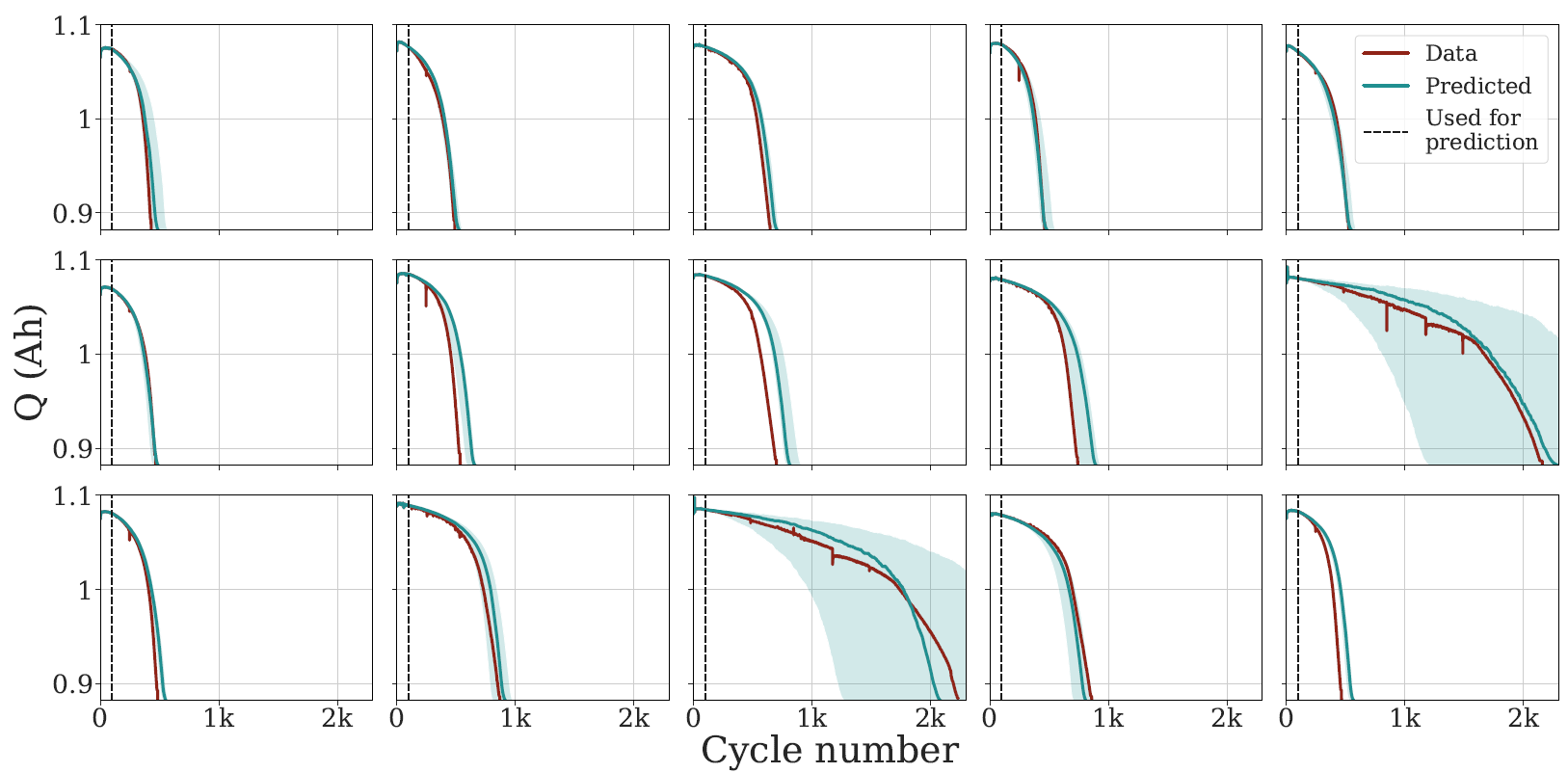
- Authors: Laura Hannemose Rieger, Eibar Flores, Kristian Frellesen Nielsen, Poul Norby, Elixabete Ayerbe, Ole Winther, Tejs Vegge and Arghya Bhowmik
- WP10 update from Helge Stein (April 2023)
- Helge Stein hosted the Baden-Württemberg prime minister Winfried Kretschmann at his laboratory back in febraury. The prime minister says "Ulm is a hotspot of which we are proud" after visiting Prof. Steins lab. Read more about the visit HIU-website and in the local newspaper.
- The autonomous lab is currently being integrated in BIG-MAP's FINALES project. FINALES is currently being tried and tested with a final run being planned for may this year.
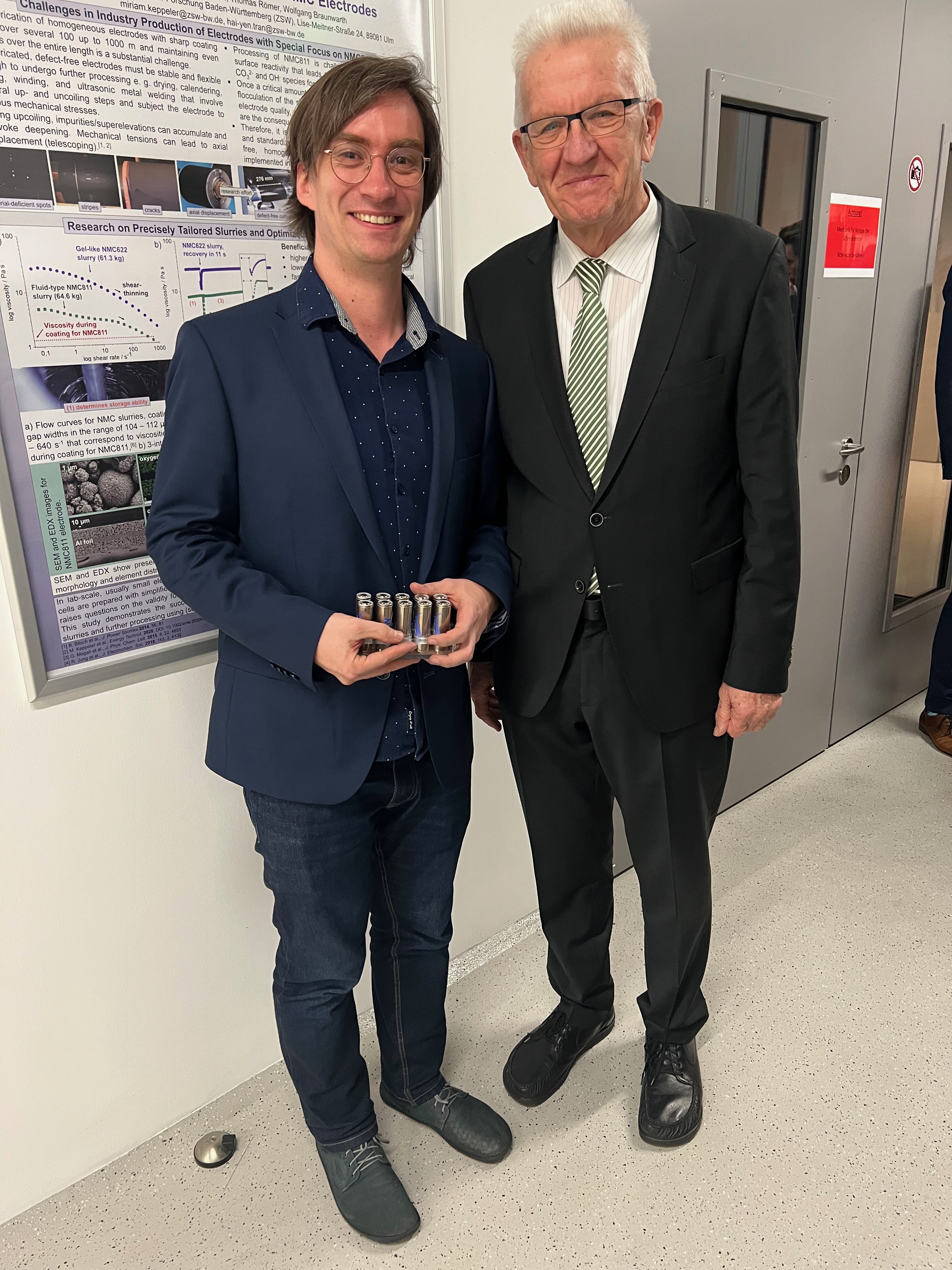
Prime Minister Winfried Kretschman is impressed with the newly setup autonomous lab by Prof. Stein
WP6 update including collaboration with WP3, WP5 and WP11 (Janaury 2023 update)
- Experimental activities focused on the final deliverable D6.5 and acquisition of reliable, pertinent datasets accross the the entire lifetime of the project defined cell chemistries containing electrolyte conducting salts and functional additives synthsized by our partners from WUT. Two research papers in pipeline: collaboration between WUT,CID, KIT and FZJ. Strong collaboration with WP3, WP5 and WP11 throught esynergistic experimental and theoretical activities.
- The overall workflow representation from experimentation to data generation in Helmholtz-Institute Münster and data analysis in Karlsruhe Institute of Technology (KIT) and its partners, seen below
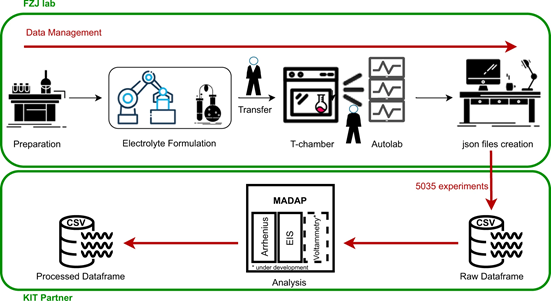
WP5 progress (Janaury 2023 update)
- The tier 2 multi-techniques experimental workflow to characterize the BIG-MAP materials and cells is currently involving all partners, focusing on investigating aged graphite/LNO electrodes prepared with a new electrolyte. The samples were cycled in standardized conditions by CIDETEC and Julich, dismounted at 80% state of health, and delivered to the various sites to be probed by XPS, 2D Raman spectroscopy, in-lab X-ray diffraction, operando synchrotron XRD, ex situ synchrotron nanotomography, operando XAS, neutron depth profiling, FIB-SEM, TEM, NMR. This combination of techniques will provide a full screening of electrodes properties and data for modelling and AI workpackages.
- Image below: Joint ESRF and CEA team effort to measure operando the lithiation mechanism in aged LNO electrode at the level of single particles by scanning x-ray diffraction microscopy on beamline ID01. Main contact : François Cadiou (ESRF) / Quentin Jacquet (CEA).
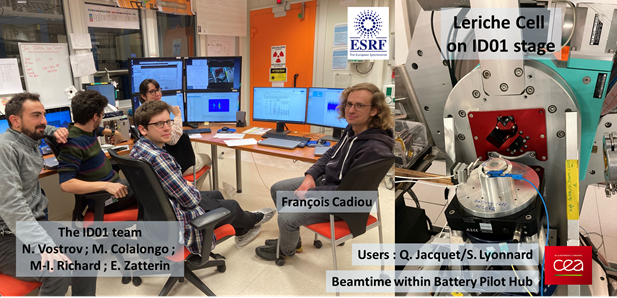
WP4 Highlights Fall 2022
The BIG-MAP robotics team arranged an on-site visit in October in Würzburg, Germany to boost the project progress towards an integrated robotic system for the synthesis of battery materials.
The participants were our partners from the IT University of Copenhagen, Rodrigo Moreno and Jonas Jensen. Ihar Suvorau joined in virtually from University of Tartu via Teams.
The meeting was organized by Shahbaz Bandesha, Fraunhofer ISC, with support from Simone Peters and Henning Lorrmann.
The face-to-face workshop had four main objectives
- Install the second prototype of the liquid-liquid-extraction (LLE) device in the central robot unit and test with relevant chemistries (silanes and ethyl acetate)
- Implement a trans-national machine-to-machine communication channel for the LLE device via an optimized PLC
- Enable historical data for important information produced by the liquid extraction device.
- Construction support in the for the extraction fume set of the robot system.
After five days filled with concentrated effort, we achieved most of our ambitious targets and made a big leap towards the final installation of the organic robotic system.
No less important, we fostered our partnership with some after-work activities and culinary highlights (burger and beer) after long working days.
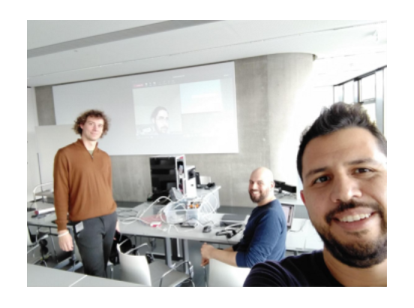
WP2 Highlights Fall 2022
The work in WP2 proceeds on the overall goal to find new modelling solutions that make computer simulations at the atomic scale more capable, more accurate and more realistic. Here ML approaches that mimic quantum-mechanical (QM) results without the explicit (and costly) presence of the electrons play a particularly important role. Among recent WP2 achievements we note:
- CNR has been working to the development of automated workflows for the ab initio simulation of x-ray spectroscopic fingerprints in support to experiments. Some basic tools have been already published on github within the official AiiDA plugin for Quantum ESPRESSO (https://github.com/aiidateam/aiida-quantumespresso), while a more complete version is expected soon. Stay tuned!
- UNIVIE and collaborators have also been making progress towards algorithmic and systematic virtual sampling of the chemical compound space of electrolytes. They have also been working on AI methods that will exploit and benefit from the integration of diverse synthetic and experimental data sets.
- New accurate ML potentials for electrolyte mixtures have been developed by CAMU in collaboration with UU. For a range of compositions and temperatures these new potentials manage to give close to perfect agreement with ab initio-MD simulation results, but at a fraction of the cost.
Congratulations to CAMU and UU for their poster "Machine Learning Force Field for Organic Liquids: the EC/EMC Binary Solvent" being awarded an honorable mention in the "Statistics and configuration sampling" symposium at the Psi-k conference in Lausanne, August 22-25, 2022 (presenter Ioan Bogdan-Magdau). This work also won the prize as "most commended poster" at the BIGMAP project meeting 7-8 September in Denmark.
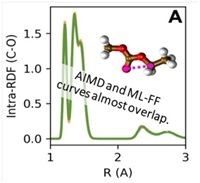
(photo: presenter Daniel Arismendi-Arrieta
and coordinator Tejs Vegge)
- WWU has successfully implemented (in cooperation with 3DS) the application of rs@MD to the intial SEI growth in LP57 at a model anode. Space-time-resolved trajectories for the SEI products are now available and are currently analysed further.