A key part of the BIG-MAP effort was focussed on automating the construction of force field models that allow the investigation of battery components (their behaviour, properties and degradation) on molecular length and time scales. Machine learning enables the distillation of expensive electronic structure calculations into fast force fields. An example of such a force field, trained only on inorganic crystal structures obtained from the Materials Project shows stable molecular dynamics simulation of a prototype interface between graphite and LP57 electrolyte (EC/EMC and LiPF6).
The first paper focussed on building up a dataset of small organic solvent models corresponding to LP57 (a mixture of ethylene carbonate (EC) and ethyl-methyl carbonate (EMC)),and fitted with one of the "first generation" machine learning (ML) force field models, specifically Gaussian Approximation Potential (GAP). Careful analysis and iterative training, lead by Ioan-Bogdan Magdau, allowed the correct reproduction of the electrolyte density under a variety of EC/EMC compositions and temperatures. Current work is ongoing on simplifying the fitting protocol, and identifying the correct level of electronic structure theory that is accurate enough to obtain not just static properties, such as the density, but dynamic properties of interest to battery design, such as diffusivity and viscosity.
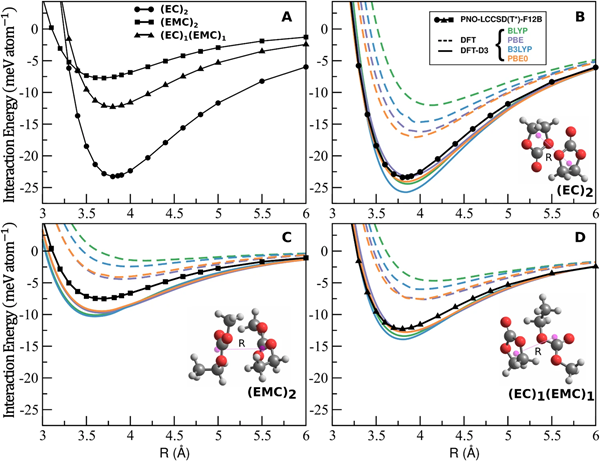
More recently, we have been working to simplify the protocol to generate force fields and in particular to make it easier to start doing molecular dynamics even before new training is attempted. This is done with so-called 'foundation models', trained to a large variety of structures, not specific to any application. The animation below (created by Cas van der Oord) shows the first attempt, using a model trained only on the inorganic crystal structures of the Materials Project, doing stable molecular dynamics of the interface between graphite and LP57. The system size is still small, and nothing terribly interesting happens in these few hundred picoseconds, but that this level of extrapolation from crystals to organic liquid interfaces is a huge milestone for model building. To help appreciate this, the next video is a reel of some of the crystal structures in the training set of the model.