BIG-MAP has developed a modular robotic synthesis platform. The vision behind the platform is to achieve the fully automated synthesis of battery materials, leveraging the power of machine learning and AI for self-driving experimental materials optimization. A specific focus has been put on the modularity of the platform both on the hardware and software level allowing for the rapid exchange of modules. This will make it flexible and extensible, which is key in a rapidly evolving research environment.
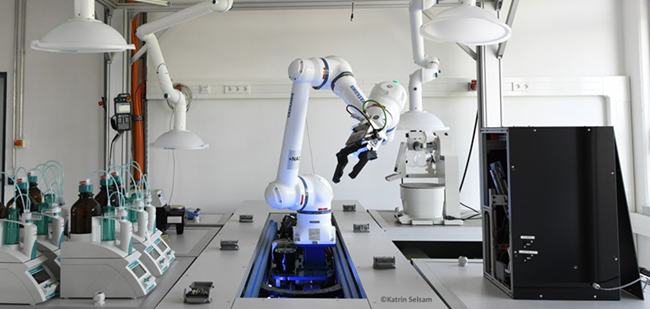
On the hardware side, the platform utilizes a device container system where each container is dedicated to a specific task. These containers can be easily exchanged using a standardized connector system for mechanical positioning, power, and data transfer. Adding or removing a container is as simple as inserting or removing a single plug.
On the software level, the platform achieves seamless orchestration of workflows through standardized interfaces implemented once for each device container. These interfaces rely on industrial protocols such as REST APIs and OPC-UA, ensuring efficient and reliable communication between the central orchestration unit and various kinds of devices that are either custom-made or retrieved from commercial vendors.
First integration tests have proven successful, showcasing the platform's capabilities in fully autonomous optimization. For example, the platform has demonstrated its ability to optimize the recipe for mixing user-defined colors from differently colored liquids, highlighting the practical application of the engineering concepts embedded in both the hardware and software. This paves the way for future integration of modules specifically designed for battery material optimization.
Furthermore, the benefits of modular robotic platforms extend beyond battery research. The methods developed by BIG-MAP are transferrable to a wide range of scientific experiments, promising a broad and impactful reach.