BIG-MAP has developed an active learning algorithm to speed up the segmentation of battery electrodes. Capturing the complex 3D microstructures can give insight about their operational properties and dynamic changes that occur during cycling. However, segmentation with the near-perfect accuracy required is a challenging task. We use a deep learning algorithm, a U-Net for segmentation and employ active learning to minimize the needed for training data.
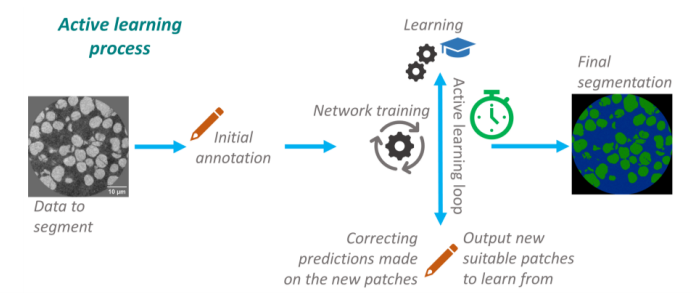
Battery electrodes have a complex 3D microstructure, with a near-random spatial organization, which in turn affects their operational properties. Moreover, during cycling, dynamic changes occur in the material’s microstructure. These changes can be investigated with non-destructive 3D imaging techniques, such as X-ray tomography, which produce large experimental datasets at each acquisition/time-step to obtain final 3D volumetric reconstructions with sufficiently high resolution. In a raw 3D volume obtained through tomography, each voxel has a value which can be linked to a material in the sample. Segmentation is the process of attributing a phase to each voxel in the raw volume.
Quantitative analysis requires the segmentation to be precise as this strongly influences the ultimate analysis precision and fidelity. Thus, segmentation of tomographic datasets for quantitative analysis is then a long and challenging process. For complex microstructures, standard segmentation algorithms tend to fall short when aiming for the highest fidelity; machine learning models can then be investigated to improve this. The best results seem to come from either highly specific algorithms or U-Net like based CNNs, both of which are very time consuming, human intensive and require specific setups.
With our algorithm we aim to substantially reduce the human annotations needed by only annotating the data that benefits the model the most. In the initial step, a small number of images are annotated roughly (greatly cutting down the time needed compared to a precise annotation). We also have a large pool of unlabeled data from which we aim to only annotate the samples that will increase the accuracy the most.
We train the model until it no longer improves with the initial data. The algorithm then surveys the unlabelled data pool and chooses the patches that will be most useful, i.e., will increase the accuracy of the model the most. It then outputs its initial guess of the segmentation to the user. The user corrects the segmentation and restarts the training. This process is repeated until either the needed accuracy is reached, or a predefined labelling budget is exhausted.
By only needing annotation for a fraction of the data and providing a suggestion for the segmentation, we can greatly reduce the time needed to annotate electrode microstructures, accelerating research and gaining new insights into how the geometric structure and microstructure of the electrode influence its behavior and are influenced by, e.g., cycling.